Artificial Intelligence (AI) is transforming the manufacturing industry, driving unmatched levels of efficiency, productivity, and innovation. Whether it’s through predictive maintenance or generative AI design, manufacturers are using AI to stay ahead in today’s fast-changing world.
But what does AI in manufacturing actually involve, and how is it shaping the future?
In this article, we’ll dive into AI’s role in manufacturing, breaking down its applications with real-world examples, and exploring the potential of generative AI. If you’re curious about how AI is revolutionizing the industry, this guide will give you the answers and key insights you need.
Introduction to AI in Manufacturing
Manufacturing has long been an industry driven by innovation. From the Industrial Revolution to the adoption of robotics, each technological leap has significantly impacted how goods are produced. Now, AI is emerging as the next frontier in manufacturing, reshaping operations across the entire supply chain.
The integration of AI technologies like machine learning, computer vision, and generative AI is enabling manufacturers to optimize processes, reduce costs, and improve quality in ways that were previously unimaginable.
With its ability to analyze vast amounts of data, predict future outcomes, and automate complex processes, AI is a powerful tool that manufacturers are eager to leverage. But how is AI being used in real-world manufacturing, and what does the future hold for this evolving technology?
Key Applications of AI in Manufacturing
Predictive Maintenance
One of the most significant applications of AI in manufacturing is predictive maintenance. Traditional maintenance schedules often rely on time-based intervals, leading to unnecessary downtime or catastrophic failures.
AI-powered predictive maintenance systems analyze data from machinery to predict when a component is likely to fail, allowing for repairs to be made just in time. For example, Siemens uses AI algorithms to monitor equipment in real-time, predicting potential failures and reducing unplanned downtime by up to 50%.
Key Benefits:
- Reduced downtime
- Lower maintenance costs
- Increased operational efficiency
Generative Design
Generative AI is a design process where AI algorithms generate numerous design options based on specified constraints, such as materials, weight, and strength. This technology is proving invaluable in industries like aerospace and automotive, where lightweight materials are crucial for performance.
For instance, General Motors partnered with Autodesk to use generative AI in designing lighter, stronger car parts. The result? A seat bracket that is 40% lighter and 20% stronger than previous designs.
Key Benefits:
- Optimized designs
- Reduced material waste
- Faster innovation cycles
Quality Control and Inspection
AI is improving quality control in manufacturing through advanced computer vision systems. Traditional inspection methods can be slow and prone to human error. AI-powered systems can analyze products on the production line in real time, identifying defects with greater accuracy and speed.
Foxconn, a major electronics manufacturer, uses AI-driven visual inspection systems to enhance the quality control of iPhones, detecting even the smallest imperfections.
Key Benefits:
- Higher quality products
- Reduced waste
- Faster inspection times
Supply Chain Optimization
Supply chain management is complex, with numerous factors like demand forecasting, inventory management, and logistics. AI-driven systems help manufacturers predict demand more accurately and optimize supply chain processes.
For example, Intel uses AI to predict supply chain disruptions and adjust production schedules accordingly, reducing lead times and avoiding stockouts.
Key Benefits:
- Improved demand forecasting
- Efficient inventory management
- Enhanced customer satisfaction
Robotics and Automation
AI-powered robotics are making manufacturing more agile and flexible. Collaborative robots, or “cobots,” can work alongside humans to perform repetitive tasks, such as assembly or packaging, reducing labor costs and increasing productivity.
BMW uses AI-powered robots to assemble car parts, significantly reducing the time required to produce each vehicle while maintaining high precision and safety standards.
Key Benefits:
- Increased productivity
- Reduced human error
- Lower labor costs
Generative AI in Manufacturing Examples
Generative AI in manufacturing is pushing the boundaries of what’s possible, allowing manufacturers to create new designs and processes that were once unimaginable. Here are some examples of how generative AI is being used:
1. Airbus – Lightweight Aircraft Components
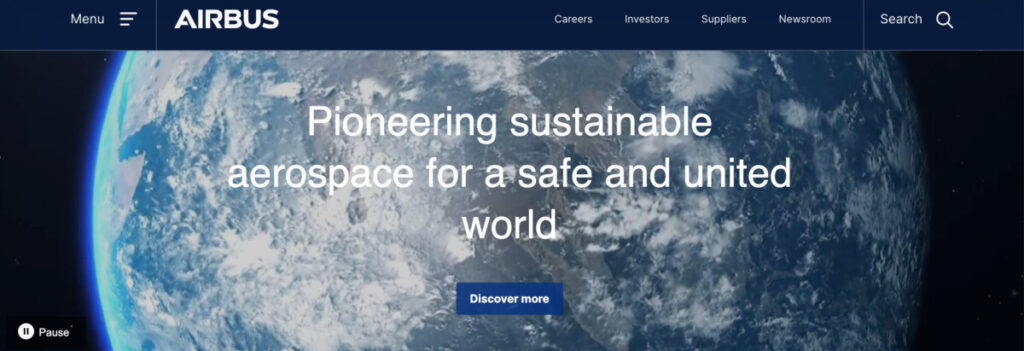
Airbus has been using generative AI to optimize the design of aircraft components. By feeding parameters like material constraints, weight, and strength into generative design algorithms, Airbus can explore thousands of design possibilities and choose the most optimal ones.
This has resulted in lighter components that use less material while maintaining or improving structural integrity. For example, Airbus used generative AI to design a partition for its A320 aircraft that is 45% lighter than previous versions.
Impact:
- Weight reduction: Helps to reduce fuel consumption, leading to lower operating costs and reduced environmental impact.
- Material savings: Minimizes waste by optimizing the use of materials, which is critical in aerospace manufacturing.
2. General Motors – Automotive Design
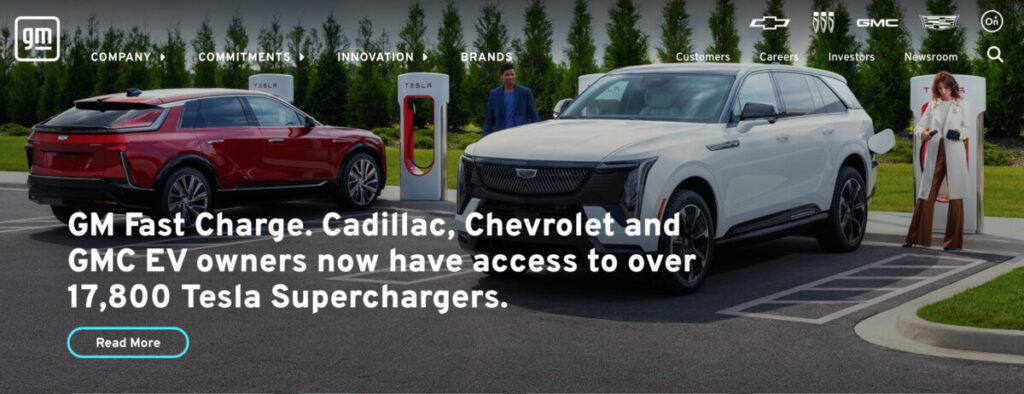
General Motors (GM) has been leveraging generative AI for automotive part design. Working with Autodesk, GM has used AI-driven algorithms to redesign components for its vehicles.
One notable success was the creation of a seat bracket that is 40% lighter and 20% stronger than its predecessor. This advancement not only reduces vehicle weight but also enhances safety and performance.
Impact:
- Fuel efficiency: Reducing vehicle weight leads to better fuel economy and reduced emissions.
- Safety improvements: AI-generated designs help improve structural integrity without adding unnecessary bulk.
3. Adidas – Sustainable Footwear
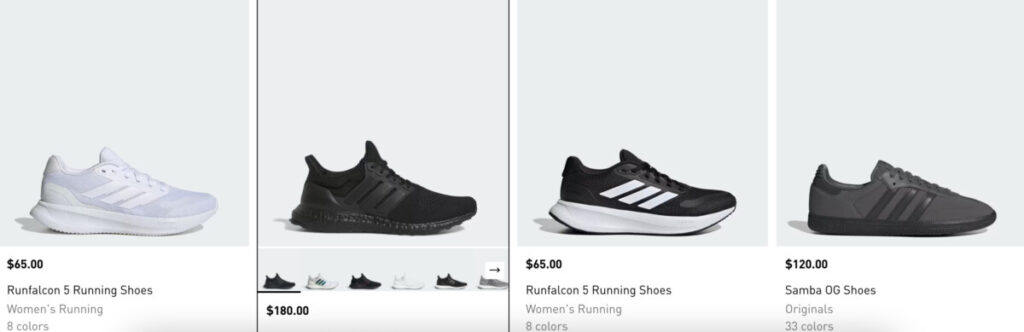
Adidas has turned to generative AI to create more sustainable and efficient footwear designs. The company uses AI to develop innovative designs that reduce material waste while maintaining product performance.
For example, Adidas partnered with Carbon, a 3D printing company, to develop its Futurecraft 4D shoes, which are designed using generative AI to optimize cushioning and performance.
Impact:
- Sustainability: AI-generated designs help Adidas reduce material use and waste, aligning with the company’s sustainability goals.
- Customization: Generative AI allows Adidas to create custom-fit shoes that are tailored to the specific needs of athletes.
4. Stanley Black & Decker – Optimized Tool Design
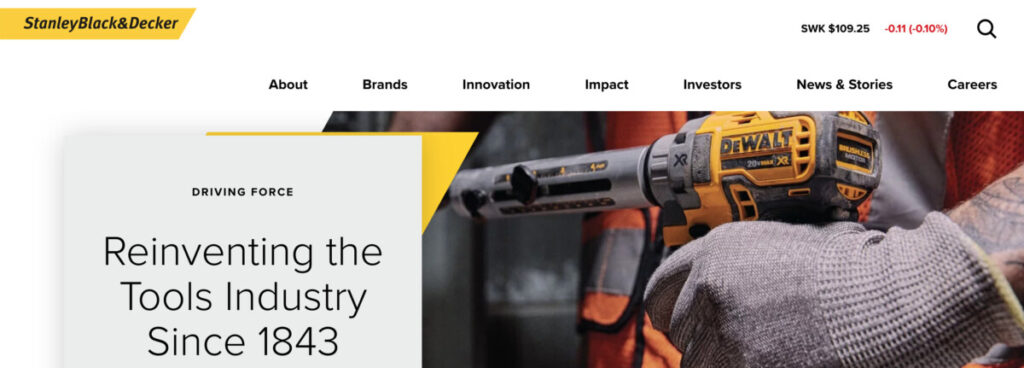
Stanley Black & Decker, a global leader in hand tools and storage, uses generative AI to optimize the design of industrial tools.
By inputting specific requirements like material strength, weight, and manufacturing constraints, the company can create more efficient tools that are lighter and stronger. Generative AI also helps the company reduce the number of prototypes needed, speeding up the design-to-production process.
Impact:
- Faster design cycles: Generative AI reduces the time spent in the prototype stage, accelerating product development.
- Material optimization: AI-generated designs minimize excess material use, reducing production costs.
5. Under Armour – Performance Sportswear
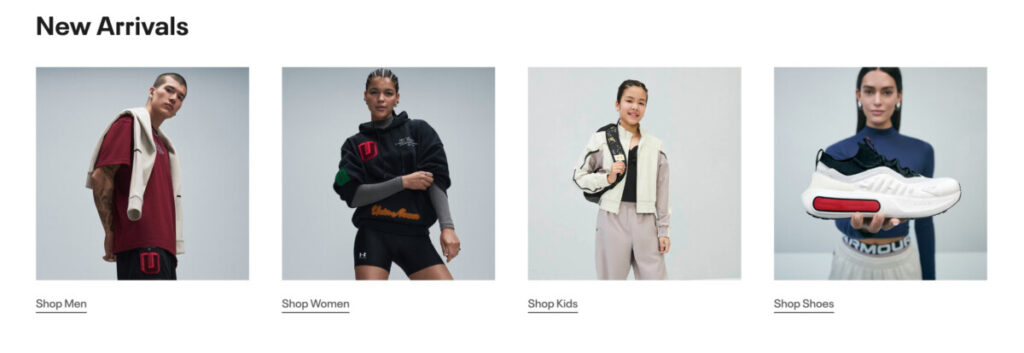
Similar to Adidas, Under Armour uses generative AI to improve the design of its sportswear products. The company leverages AI to create designs that enhance the performance of athletes by optimizing features like breathability, flexibility, and strength. This approach has resulted in more efficient manufacturing processes and reduced material waste.
Impact:
- Enhanced performance: AI helps Under Armour design sportswear that improves athletic performance by fine-tuning factors such as weight and fit.
- Sustainability: Generative AI aids in reducing material waste during production, helping the company meet sustainability targets.
6. Boeing – Generative AI for Aircraft Parts
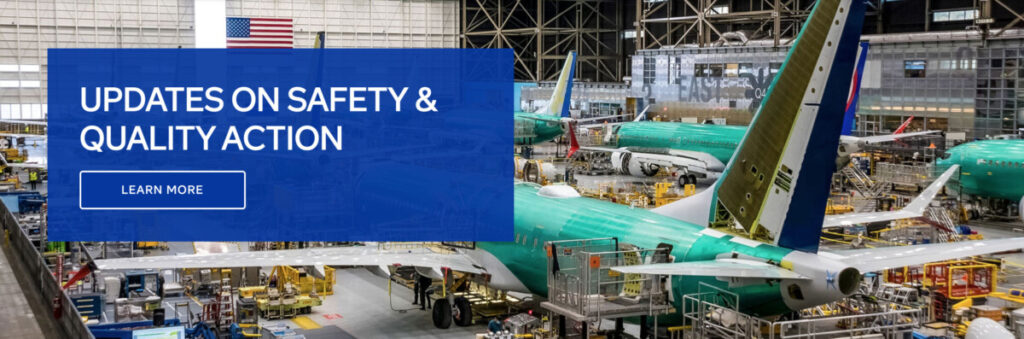
Boeing has also embraced generative AI in its manufacturing processes. By using AI to design parts for its aircraft, Boeing has been able to create lighter and more efficient components.
This approach is particularly valuable in the aerospace industry, where weight reduction translates directly into fuel savings and lower emissions. Boeing uses AI to analyze multiple design iterations, selecting the most efficient configurations.
Impact:
- Weight reduction: Lighter components lead to more fuel-efficient aircraft.
- Design innovation: AI allows Boeing to explore complex, organic shapes that are impossible to design manually.
7. Furniture Manufacturing – Customizable Designs
Generative AI is making its way into furniture manufacturing, where it is used to create customizable, efficient designs. Furniture companies are using AI to optimize the use of materials, reduce waste, and create designs that are not only functional but also aesthetically pleasing.
This technology is particularly useful in designing furniture that meets specific customer requirements, such as adjustable or modular designs.
Impact:
- Customization: AI enables the creation of bespoke furniture designs tailored to individual customer needs.
- Sustainability: AI helps reduce material waste, contributing to more sustainable manufacturing processes.
8. Nike – AI-Driven Shoe Design
Like Adidas, Nike is using generative AI to innovate in shoe design. Nike’s research teams use AI to explore new materials and designs that enhance performance, durability, and sustainability.
By feeding specific performance data into AI algorithms, Nike can create shoes that are optimized for different sports, reducing the need for extensive physical prototyping.
Impact:
- Performance optimization: AI-generated designs improve the performance characteristics of Nike shoes, such as support and flexibility.
- Rapid prototyping: AI reduces the number of physical prototypes required, speeding up the development process.
Factors Driving the Adoption of AI in Manufacturing
1. Increased Efficiency and Productivity
AI enables manufacturers to automate repetitive tasks, optimize production processes, and enhance overall operational efficiency. By deploying AI-powered systems, companies can increase the speed and precision of manufacturing tasks, reduce errors, and ensure consistent quality in production.
- Example: AI-driven robots can work alongside human workers to automate tasks such as assembly, welding, and packaging. This reduces the time taken to complete these tasks and minimizes human error.
2. Cost Reduction
One of the primary motivations for adopting AI in manufacturing is cost reduction. AI-powered systems can analyze data to find inefficiencies, optimize resource usage, and lower production costs. Additionally, predictive maintenance, enabled by AI, reduces downtime by forecasting equipment failure before it happens.
- Example: By predicting equipment malfunctions, AI systems can schedule maintenance in advance, thus avoiding costly production delays.
3. Improved Quality Control
AI enhances quality control in manufacturing by detecting defects and anomalies during production. Machine learning algorithms analyze real-time data from sensors and cameras to identify issues that may not be visible to human inspectors.
This ensures that defective products are caught before they reach the consumer, leading to better customer satisfaction and lower recall rates.
- Example: AI-based visual inspection systems are used in automotive manufacturing to identify flaws in car parts, such as scratches or misalignments, at an early stage of production.
4. Customization and Flexibility
AI allows manufacturers to offer more customized products and services. By analyzing consumer data, AI can help design products that meet specific customer needs. Additionally, generative AI enables manufacturers to explore numerous design possibilities, leading to innovative product designs that are tailored to customer preferences.
- Example: AI-driven customization platforms enable companies to offer products with personalized features, such as customizable footwear or automotive interiors, without increasing production costs.
5. Supply Chain Optimization
AI helps manufacturers optimize their supply chains by forecasting demand, managing inventory, and optimizing delivery routes. AI-powered tools can process vast amounts of data to identify patterns and trends that influence demand, leading to better production planning and inventory management.
- Example: AI-driven supply chain solutions can predict material shortages and delays, allowing manufacturers to adjust production schedules and avoid stockouts or overproduction.
6. Sustainability and Waste Reduction
Environmental sustainability is an increasingly important focus for manufacturers. AI can help reduce waste by optimizing resource usage, minimizing material waste, and reducing energy consumption. AI algorithms can analyze production processes to identify areas where materials and energy are being wasted and suggest improvements.
- Example: Generative AI in manufacturing can optimize product designs to use fewer materials without compromising strength or quality, resulting in reduced waste during production.
7. Labor Shortages and Workforce Augmentation
The manufacturing industry faces labor shortages in various regions, particularly for skilled workers. AI-powered robots and automation systems can help bridge this gap by performing repetitive and physically demanding tasks.
This allows human workers to focus on more complex and creative aspects of manufacturing, such as product design and process improvement.
- Example: AI-powered cobots (collaborative robots) can assist human workers in tasks like material handling and machine operation, reducing the physical strain on workers and increasing productivity.
8. Predictive Maintenance and Reduced Downtime
AI enables predictive maintenance by continuously monitoring equipment and analyzing data to predict when machines are likely to fail. This reduces the likelihood of unexpected breakdowns, which can be costly and time-consuming. Predictive maintenance helps manufacturers keep production lines running smoothly and efficiently.
- Example: AI systems in factories can monitor temperature, pressure, and vibration in machinery to predict when a component is likely to fail, allowing for timely maintenance and reducing downtime.
9. Faster Decision Making
AI-driven analytics platforms provide manufacturers with real-time insights into production data. These insights enable faster and more informed decision-making, allowing manufacturers to respond quickly to changing market conditions, supply chain disruptions, or equipment malfunctions.
- Example: AI-powered dashboards can display key performance indicators (KPIs) in real-time, helping plant managers make quick adjustments to optimize production.
10. Innovation and Competitiveness
Companies adopting AI in manufacturing are often at the forefront of innovation. The ability to use AI to optimize processes, improve product designs, and enhance customer experiences gives these companies a competitive edge in the marketplace.
Early adopters of AI in manufacturing are more likely to lead their industries and differentiate themselves from competitors.
- Example: Companies that use AI to drive innovation, such as Tesla with its AI-driven assembly lines, have been able to maintain a strong competitive advantage in their respective industries.
Future of AI in Manufacturing
The future of AI in manufacturing looks bright, with more innovations on the horizon. As AI technologies like machine learning and generative design continue to mature, manufacturers will have even more tools at their disposal to enhance their operations.
Some key trends to watch include:
- AI-Powered Smart Factories
AI will enable fully autonomous factories where machines and robots collaborate seamlessly with minimal human intervention. - AI-Driven Sustainability
Manufacturers will increasingly use AI to create sustainable production processes, reducing carbon footprints and minimizing waste. - AI-Enhanced Human-Machine Collaboration
AI will continue to empower workers by handling repetitive tasks, allowing them to focus on creative and strategic decision-making.
The Bottom Line
AI is no longer a futuristic concept in manufacturing—it’s here, and it’s transforming the industry. As we look to the future, the role of AI in manufacturing will only grow, leading to smarter, more efficient factories.
Manufacturers that embrace AI today will be well-positioned to lead the industry tomorrow. The time to invest in AI is now, as the technology offers unprecedented opportunities to streamline processes, cut costs, and drive sustainable growth.
If you’re looking to stay ahead of the curve in the manufacturing world, AI is the key to unlocking your company’s potential.
FAQs
1. How is AI used in manufacturing?
AI is used in manufacturing to improve automation, optimize production lines, predict equipment failures, ensure quality control, and reduce downtime. AI-driven robots and smart systems help streamline processes, detect defects, and manage supply chains more efficiently.
2. How is AI being used in production?
In production, AI enhances efficiency by automating repetitive tasks, improving machine performance through predictive maintenance, and optimizing workflows. AI can also analyze data in real time, improving decision-making and production outcomes.
3. Which company uses AI in manufacturing?
Several companies use AI in manufacturing, including General Electric (GE), Siemens, BMW, and Toyota. These firms employ AI to optimize operations, enhance product quality, and increase production efficiency.
4. What is AI in manufacturing’s advantages and disadvantages?
Advantages: AI improves efficiency, reduces operational costs, enhances product quality, enables predictive maintenance, and supports real-time decision-making.
Disadvantages: High initial costs, potential job displacement, data privacy concerns, and the need for skilled personnel to manage AI systems are some drawbacks.