Today’s AI-powered BI platforms do more than report on the past—they interpret context, forecast trends, and adapt to new data in real time. This shift is not just technological; it’s transformational.
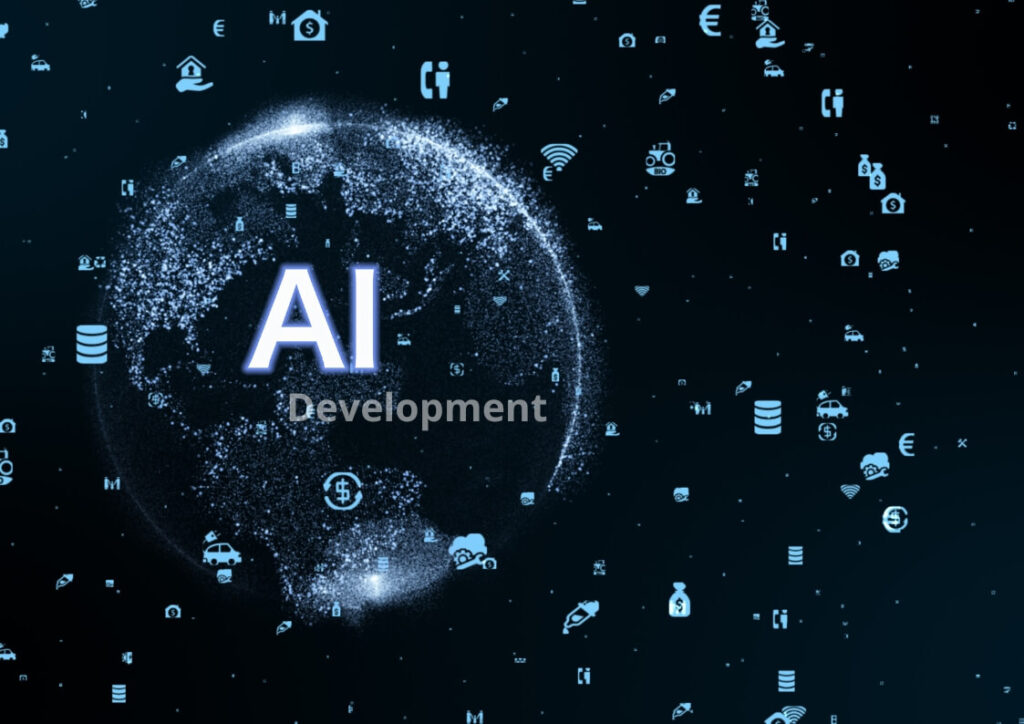
Enterprises embracing AI development in business intelligence are gaining the tools to lead in competitive, data-driven markets. In this article, we’ll explore the strategic value of AI in enterprise applications, break down its core use cases, and provide practical guidance for organizations ready to embrace AI-enhanced BI.
Why AI Is a Game-Changer for Modern Business Intelligence Systems
Artificial intelligence is ushering in a new era for business intelligence—one where data doesn’t just inform but empowers. By embedding AI capabilities such as machine learning and natural language processing into BI tools, businesses are transitioning from reactive reporting to proactive, insight-driven decision-making.
From Reporting to Predictive Insights – A New Era of Business Intelligence
Traditional BI platforms primarily focused on descriptive analytics—offering historical reports and static dashboards. While valuable, these systems often left decision-makers with more questions than answers. Enter AI: through AI development in business intelligence, organizations can now uncover hidden patterns, detect anomalies, and anticipate future outcomes with high accuracy.
AI-driven BI empowers users to:
- forecast business trends based on real-time and historical data;
- simulate “what-if” scenarios using advanced predictive models;
- identify actionable insights autonomously, reducing time to decision.
How Machine Learning and NLP Enhance Data Interpretation
Machine learning algorithms continuously learn from new data inputs, improving their predictions over time without explicit programming. This self-improvement enables BI tools to evolve alongside the business itself. For instance, ML models can automatically segment customer behavior, detect fraud patterns, or optimize inventory management based on shifting demand.
At the same time, natural language processing (NLP) simplifies data interaction. Instead of writing complex queries, users can ask questions in plain English—“What was our highest-performing region last quarter?”—and receive instant, data-backed answers. This democratizes access to analytics and minimizes dependency on technical teams.
The Shift from Historical to Real-Time Analytics
In the age of instant gratification, decision-making can no longer afford delays. AI enables real-time analytics by processing data streams as they are generated—from IoT devices, CRM platforms, financial systems, and beyond.
With AI in enterprise applications, leaders gain immediate visibility into operational performance, customer behavior, and market dynamics. This agility allows companies to:
- react to changes the moment they occur;
- adjust strategies dynamically;
- personalize customer experiences at the moment.
AI in Enterprise Applications: Key Use Cases Driving Innovation
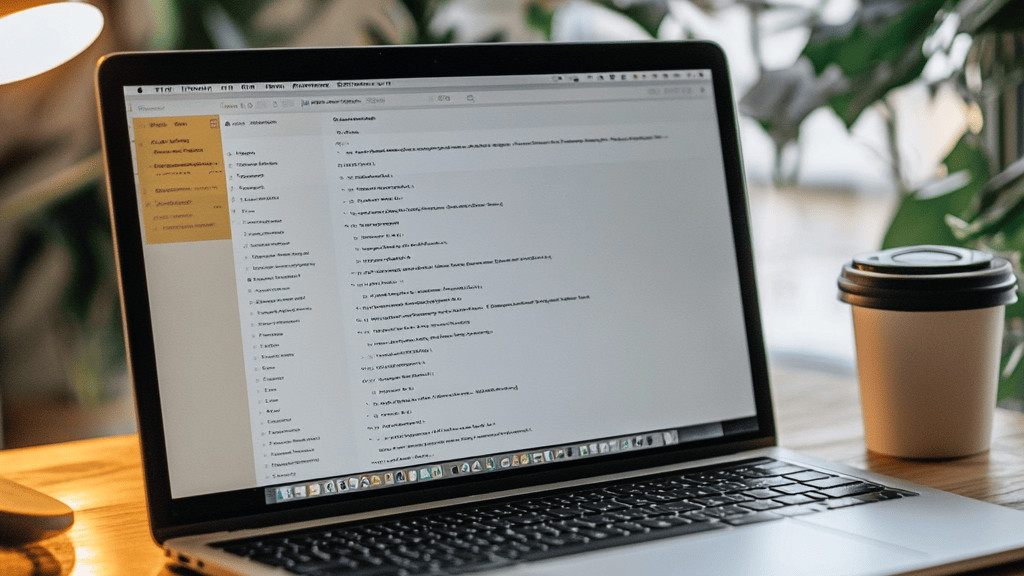
Beyond dashboards and charts, AI is fueling a revolution in enterprise-wide intelligence. Modern organizations are increasingly embedding AI into their BI ecosystems to unlock automation, enhance forecasting accuracy, and make smarter, faster decisions. These applications are reshaping the way businesses operate—from supply chains to customer relationships.
Forecasting Market Trends and Consumer Demand with AI
One of the most impactful uses of AI in BI is its ability to predict market shifts and customer needs. By analyzing historical sales data, external economic indicators, and behavioral trends, AI models can anticipate fluctuations in demand and guide proactive decision-making.
AI-Driven Decision Support in Finance, HR, and Operations
AI is no longer confined to IT departments. In finance, machine learning algorithms analyze spending patterns to detect anomalies, reduce risk, and ensure compliance. HR teams use AI to forecast workforce needs, automate candidate screening, and track employee engagement.
In operations, AI helps optimize logistics, monitor equipment health, and streamline procurement. These use cases illustrate how AI in enterprise applications is not only enhancing operational efficiency but also transforming how leaders plan and execute across departments.
The Strategic Benefits of AI Development in Business Intelligence
As competition intensifies across industries, data-driven organizations are turning to AI to sharpen their competitive edge. The strategic integration of AI into BI platforms doesn’t just improve reporting—it redefines how businesses operate, plan, and grow. From cost savings to strategic foresight, the benefits are wide-ranging and long-lasting.
Improved Agility and Responsiveness in Dynamic Markets
By automating data analysis and scenario modeling, organizations can:
- respond to market changes instantly,
- identify risks before they escalate,
- seize emerging opportunities faster than competitors.
This agility is especially valuable in sectors like e-commerce, finance, and logistics, where responsiveness directly impacts revenue and reputation.
Cost Efficiency Through Intelligent Automation and Resource Allocation
Another major advantage of AI development in business intelligence is operational efficiency. AI algorithms can automate routine data preparation, reporting, and analysis—freeing up teams to focus on high-value tasks like strategic planning and innovation.
For example, an AI-enabled BI system can automatically flag underperforming marketing campaigns, suggest optimized budget reallocations, and track real-time performance—without needing daily manual input.
Enhancing Strategic Planning Through Advanced Scenario Modeling
AI takes strategic planning to a new level by enabling advanced simulations and scenario modeling. Executives can test various business strategies under different conditions and evaluate potential outcomes before making critical decisions.
This capability empowers leadership teams to:
- make more informed, data-backed strategic choices,
- prepare for disruptions with contingency planning,
- align resources with long-term goals and trends.
How to Successfully Implement AI-Enhanced Business Intelligence in Your Organization
Integrating AI into business intelligence doesn’t happen overnight. It requires a well-thought-out strategy that balances data readiness, technology alignment, and the right partnerships. Organizations that succeed in this transition don’t just adopt new tools—they build intelligent ecosystems designed for long-term agility and innovation.
Building the Right Data Foundation for AI Integration
A strong data infrastructure is essential for any AI initiative. AI models rely on clean, consistent, and comprehensive data sets to generate meaningful insights. That’s why the first step in implementation should focus on auditing and preparing your data.
Choosing Scalable Tools and Platforms with AI Capabilities
Investing in the right tools ensures your business intelligence efforts will scale as your needs evolve. Look for platforms that offer:
- native AI and ML features (such as automated forecasting, clustering, or anomaly detection),
- integration with cloud services and data lakes,
- user-friendly interfaces with NLP capabilities for non-technical teams
Leveraging External AI Development Partners and BI Specialists
Given the complexity and pace of innovation in this space, many organizations benefit from working with experienced external partners. Collaborating with a specialist in AI in enterprise applications brings valuable industry knowledge, implementation expertise, and best practices for change management.