Multimodal AI, the convergence of multiple senses in artificial intelligence, is revolutionizing the field of AI. By leveraging various modalities such as images, text, audio, and video, multimodal AI models can build more accurate and comprehensive AI systems.
Unlike unimodal models that focus on analyzing and processing one type of data, multimodal models combine information from different sources to gain a more nuanced understanding of the underlying data. This ability to integrate multiple senses enables multimodal AI to achieve smarter solutions in various industries.
From healthcare and automotive to web search and robotics, the applications of multimodal AI are vast and diverse. By exploring and integrating the senses, multimodal AI has the potential to transform how we live, work, and do business.
Understanding Multimodal AI
Multimodal AI is a subset of artificial intelligence that leverages multiple modalities, such as images, text, audio, and video, to build more accurate and comprehensive AI models. It involves integrating different types of data inputs to create a more holistic understanding of the underlying data. By considering information from multiple sources, multimodal AI models can perform various tasks, including text-to-image generation, visual question answering, and robotics navigation.
The concept of multimodal AI is rooted in the convergence of senses in artificial intelligence. Just like humans rely on multiple senses to perceive and understand the world, multimodal AI models integrate and analyze data from different modalities to gain more context and make more accurate predictions. This convergence of senses allows AI systems to have a broader scope of data compared to unimodal AI models, which are built to analyze and process only one type of data.
Types of Multimodal AI Models
There are different types of multimodal AI models, each with its own capabilities and advantages. One example is generative AI models, which have gained significant attention in recent years. Generative AI models, such as OpenAI’s DALL-E, can generate new content, such as text or images, based on existing data. These models learn patterns and relationships from multimodal data inputs and can create unique and realistic outputs.
Another type of multimodal AI model is fusion-based models, which combine information from different modalities to make predictions or classifications. These models use fusion mechanisms, such as late fusion or early fusion, to integrate and analyze data from various sources. Fusion-based models have the advantage of providing a more comprehensive understanding of complex, multi-modal information.
Real-world Examples of Multimodal AI Models
Real-world examples of multimodal AI models in action include:
- Visual question answering systems
- Language translation with visual context
- Image captioning
- Gesture recognition
- Video summarization
These applications demonstrate how multimodal AI can enhance our interactions with technology and enable more intuitive and accurate outputs. By leveraging the convergence of senses, multimodal AI models have the potential to revolutionize various industries and processes.
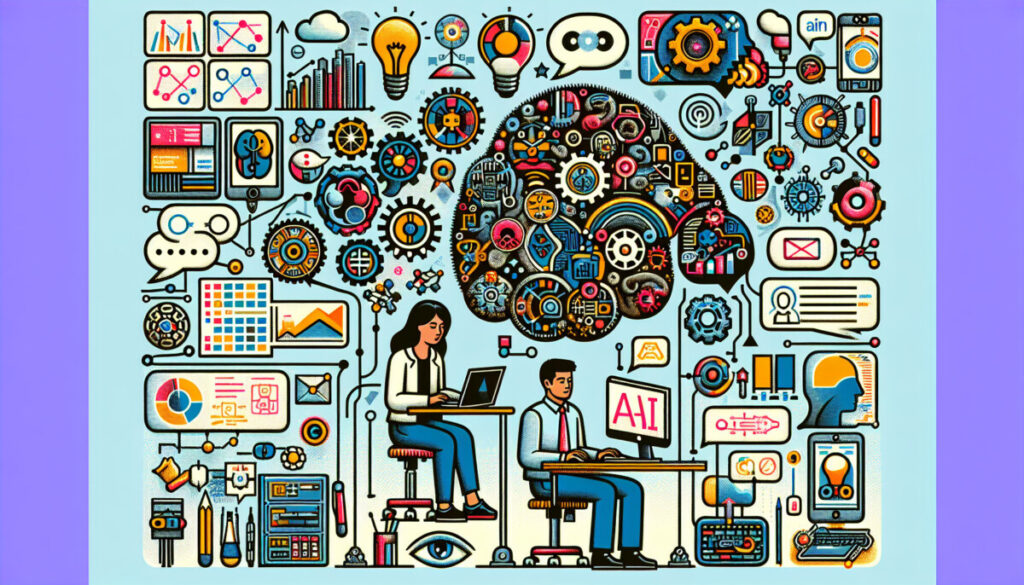
What are Multimodal AI Models?
Multimodal AI models are a subset of artificial intelligence that leverage multiple modalities, such as images, text, audio, and video, to build more accurate and comprehensive AI models. These models can combine information from different sources to create a more accurate and comprehensive understanding of the underlying data.
Compared to unimodal models that analyze and process one type of data, multimodal models have a broader scope of data, enabling them to handle context-intensive tasks more effectively by integrating and analyzing multiple modalities to gain more context.
How do Multimodal AI Models Work?
The concept of multimodal AI is different from generative AI. While generative AI focuses on creating something new from existing data, multimodal AI focuses on integrating information from different modalities to form a more complete understanding of the input data.
Multimodal AI models can improve accuracy by capturing a more nuanced understanding of the data and facilitate natural interactions between users and machines by combining different modalities like gesture and voice recognition.
Applications of Multimodal AI
As the field of AI continues to evolve, multimodal AI has the potential to revolutionize how we live, work, and do business. Its applications span various industries, including healthcare, automotive, and web search.
In healthcare, multimodal AI can help with accurate diagnoses, personalized treatment plans, and drug discovery. In the automotive industry, it is used in human-machine interface assistants, driver assistance systems, and driver monitoring systems.
By leveraging the power of multiple modalities, multimodal AI models can significantly improve business processes and customer satisfaction.
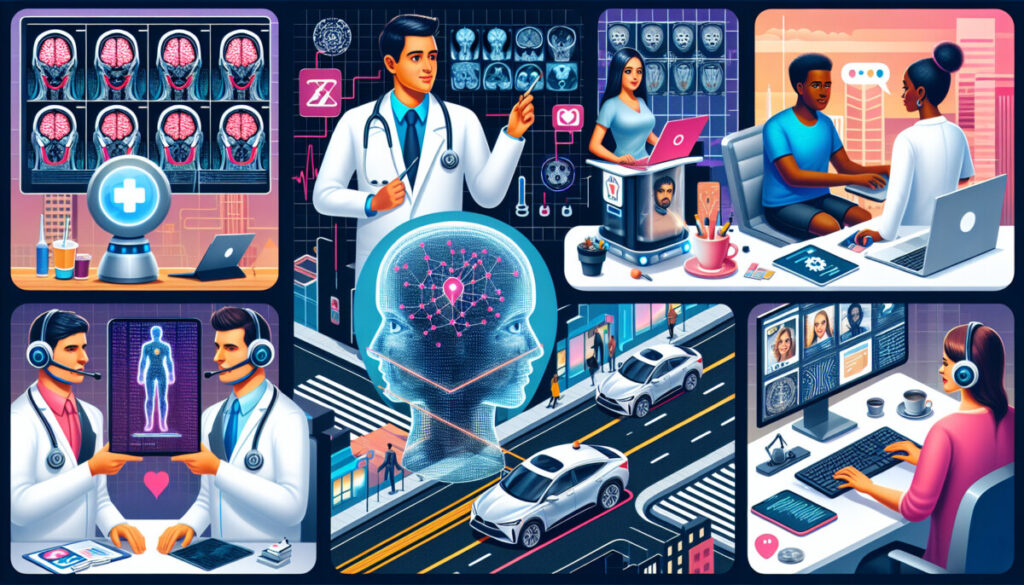
Multimodal AI models offer a more comprehensive understanding of data by integrating multiple modalities. They have the potential to revolutionize various industries and improve accuracy in tasks that require context-intensive analysis.
By embracing multimodal AI, businesses can make more informed decisions, enhance customer experiences, and drive innovation. As the capabilities of multimodal AI continue to advance, we can expect to see even more sophisticated models that can process and integrate a broader spectrum of data types.