A pressing concern is shadowing the progress of artificial intelligence: AI gender bias.
Let’s talk about that for a moment, shall we?
You already know how AI is reshaping industries, economies, and societies at an unprecedented pace. From helping doctors diagnose diseases to predicting financial trends, AI has transcended traditional boundaries, promising efficiency, accuracy, and advancement.
Yet, amidst the awe-inspiring potential of AI, it is important to understand the underlying biases that have found their way into these technologies.
In this article, we’ll explore the ins and outs of AI gender bias – examples, impacts, and proactive solutions to mitigate its adverse effects.
Understanding Gender Bias in AI
AI algorithms, though designed to be impartial, can inherit biases from their creators and datasets.
Gender bias in AI refers to the phenomenon where artificial intelligence systems exhibit preferences, prejudices, or stereotypes based on gender. This bias can manifest in various AI applications, including Natural Language Processing (NLP) systems, facial recognition software, and hiring algorithms.
At its core, AI gender bias arises from two primary sources: the biases present in the datasets used to train AI models and the biases of the individuals involved in developing and deploying these models.
1. Dataset Biases
AI systems learn from vast amounts of data, and the quality and diversity of this data significantly influence the performance and behavior of the resulting models.
Unfortunately, many datasets used to train AI algorithms are not representative of the diverse range of human experiences and identities.
For example, if a facial recognition dataset predominantly consists of images of lighter-skinned individuals, the algorithm may perform poorly when tasked with recognizing the faces of darker-skinned individuals.
Similarly, if an NLP model is trained on text primarily authored by men, it may inadvertently reinforce gender stereotypes in its language generation.
2. Developer Biases
The individuals involved in developing AI algorithms, from data scientists to software engineers, may unconsciously inject their own biases into the design and implementation process.
These biases can stem from societal norms, cultural influences, personal experiences, and implicit prejudices.
For instance, a developer may unintentionally encode gender stereotypes into an AI-driven hiring tool by training it on historical hiring data that reflects biased decision-making processes.
Similarly, the choice of language and design elements in virtual assistants may reflect and perpetuate gender norms and expectations.
AI Gender Bias Examples
1. Translation Bias
AI translation systems are good examples of gender bias in AI. They may perpetuate gender stereotypes by associating certain professions or roles with specific genders.
For instance, terms like ‘nurse’ might be consistently translated as female-gendered, while ‘doctor’ might be translated as male, reinforcing traditional gender norms and expectations.
2. Dataset Bias in Hiring
Amazon’s automated recruitment tool came under scrutiny for favoring male candidates over female candidates. This bias stemmed from the training data, which predominantly consisted of resumes from male applicants.
Consequently, the AI system learned to prioritize male candidates, perpetuating gender disparities in hiring processes.
3. Natural Language Processing (NLP)
Another AI gender bias example is in natural language processing (NLP) models. Studies have shown that these models often exhibit stereotypical associations, such as linking certain occupations or traits more strongly with one gender over another.
NLP models employed in virtual assistants like Amazon’s Alexa and Apple’s Siri are good examples of AI gender bias in NLP.
They have exhibited gender bias by associating certain professions or roles with specific genders. For example, they might correlate ‘man’ with ‘doctor’ and ‘woman’ with ‘nurse’, reflecting outdated societal stereotypes and biases.
4. Voice Assistants
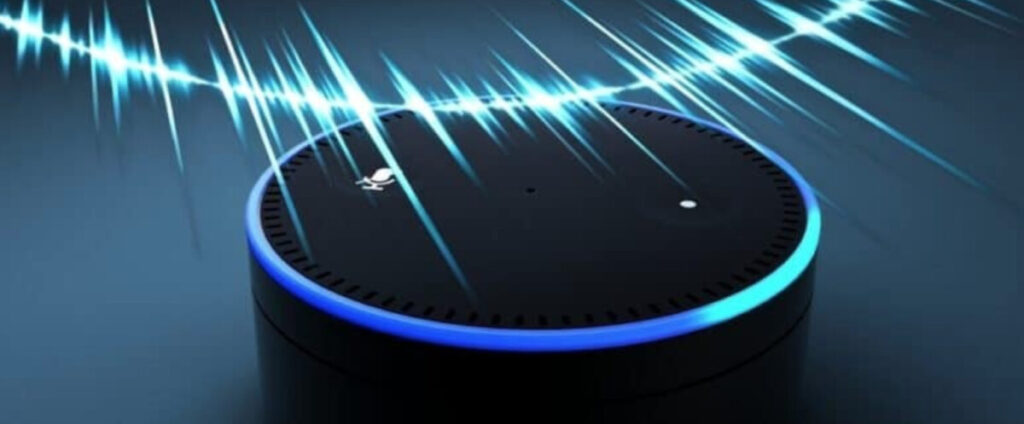
Just like we have AI gender bias in NLP, we also have it in voice assistants.
Virtual assistants like Amazon’s Alexa and Apple’s Siri were initially designed with default feminine voices, reinforcing stereotypes of women as submissive or nurturing.
This design choice contributes to the promotion of sexism in AI, shaping users’ perceptions of gender roles in technology and society.
5. Image Recognition Bias
AI image recognition software has demonstrated gender bias by displaying a disproportionately small percentage of women when prompted to identify images of professions like ‘judges’.
Also, younger women are frequently depicted in non-specialized roles, while older men are portrayed in specialized professions, perpetuating biases and assumptions about age and gender roles in society.
This bias reflects and worsens existing gender disparities in certain fields, promoting underrepresentation and inequality.
The Impact of Sexism in AI
The consequences of gender bias or sexism in AI extend far beyond mere algorithmic errors. They permeate societal structures, impacting individuals’ access to opportunities, decision-making processes, and overall well-being.
1. Limiting Access to Opportunities
AI-powered hiring tools that exhibit gender bias can severely limit women’s access to employment opportunities.
When algorithms favor male candidates due to biased training data or flawed decision-making processes, qualified female applicants may be unfairly overlooked or discriminated against. This will, of course, promote gender disparities in the workforce.
2. Marginalization in Various Domains
Biased language models used in communication platforms or content-generation tools can contribute to the marginalization of certain genders in various domains.
For example, if an NLP model consistently associates specific occupations or personality traits with one gender, it reinforces stereotypes and undermines the visibility and recognition of individuals who do not conform to these norms.
3. Amplification in Critical Sectors
The widespread deployment of AI in critical areas has amplified the potential for gender-based discrimination. Areas like healthcare, finance, and criminal justice!
Biased AI models in healthcare diagnosis may result in differential treatment or misdiagnosis based on gender, worsening health disparities.
In finance, biased algorithms can perpetuate gender pay gaps or limit access to financial services for certain demographics.
In criminal justice, biased predictive policing algorithms may unfairly target or disproportionately penalize specific genders, perpetuating systemic inequalities.
4. Loss of Trust and Confidence
Instances of gender bias in AI erode public trust and confidence in technology and its applications.
When people experience or perceive discrimination or unfair treatment by AI systems, it undermines their faith in the neutrality and objectivity of these technologies.
The loss of trust not only impacts individual interactions with AI but also prevents broader societal acceptance and adoption of AI-driven solutions.
5. Reinforcement of Gender Inequality
Perhaps most significantly, gender bias in AI causes existing gender inequalities to continue.
By replicating and amplifying societal biases and stereotypes, biased AI systems contribute to the normalization of discriminatory practices and attitudes.
This reinforcement of gender inequality not only harms individuals directly affected by biased algorithms but also undermines efforts to achieve gender equality and social justice.
Strategies for Mitigating AI Gender Bias
So how do we stop sexism in AI?
Sure, there are already multiple studies attempting to answer this question. And that’s because addressing AI gender bias requires a multifaceted approach that includes data diversification, inclusive development practices, ethical frameworks, and user empowerment.
Let’s explore these strategies in more detail:
1. Diversify Data and Algorithms
Ensuring diversity in the data used to train AI models will be super helpful in mitigating sexism in AI. This involves actively seeking out and incorporating datasets that represent a wide range of gender identities and experiences.
By training AI models on diverse datasets, developers can reduce the risk of perpetuating stereotypes and biases.
Also, algorithms should be carefully scrutinized for potential biases. Techniques such as algorithmic auditing can help identify and address gender-based disparities in AI decision-making processes.
When developers analyze the behavior of AI models, they can uncover biases and take corrective action to promote fairness and equity.
2. Foster Inclusive AI Development
Promoting diversity and inclusion within the AI development community is essential for addressing gender bias.
Initiatives aimed at encouraging the participation of women and individuals from other underrepresented groups in AI design, development, and deployment can help ensure that diverse perspectives are incorporated into the process.
Mentorship programs, targeted recruitment efforts, and the creation of inclusive work environments are also key strategies. We can create technologies that better serve the needs of all users by empowering individuals from diverse backgrounds to contribute to AI innovation.
3. Create Ethical AI Frameworks and Governance
The establishment of robust ethical frameworks and governance structures is super important for mitigating gender bias in AI.
These frameworks should outline clear guidelines and principles for the responsible development and deployment of AI systems, with a focus on fairness, non-discrimination, and the protection of human rights.
Policymakers and regulatory bodies play a crucial role in implementing these frameworks and ensuring that AI systems are held accountable for their impact on individuals and society. Yes, particularly in terms of gender-based discrimination.
By enforcing ethical standards and promoting transparency, policymakers can help foster trust in AI technologies and mitigate the risk of bias.
4. Educate and Empower Users
Educating end-users about the potential for gender bias in AI systems is essential for promoting awareness and empowerment. The result? People will become more informed consumers and advocates for more inclusive AI technologies.
Developing educational materials, training programs, and user-friendly tools can help empower individuals to critically evaluate the AI systems they interact with and advocate for fairness and equity.
The Bottom Line
Tackling gender bias in AI isn’t a one-person job. It’s going to take a village – researchers, policymakers, industry leaders, and everyday folks like us. But together, we can make strides.
By putting diversity, ethics, and user empowerment front and center, we pave the way for AI systems that truly reflect the richness of our society. I’m talking about systems that treat everyone fairly, regardless of gender.
It’s important to stay on our toes and tackle gender bias in AI head-on. Only then can we unlock AI’s full potential for the benefit of everyone, no matter who they are or how they identify.
Cheers to an AI future that leaves no one behind!
FAQS
1. Is there a gender bias in AI?
Yes, gender bias in AI is a recognized issue where AI systems can exhibit preferences, stereotypes, or prejudices based on gender, leading to unfair treatment or outcomes.
2. Is ChatGPT gender bias?
ChatGPT, like other AI systems, is not inherently biased, but it can reflect biases present in its training data or the language used by its users. Efforts are made to mitigate bias during development and training.
3. What is an AI bias example?
An example of AI bias is when a hiring algorithm favors male candidates over female candidates due to biases in the training data. Of course, this leads to gender discrimination in employment decisions.
4. Does AI advance gender equality?
AI has the potential to advance gender equality by automating tasks, facilitating access to information, and providing new opportunities. However, without careful consideration and mitigation of biases, AI can also perpetuate or exacerbate existing gender inequalities.
5. What are the biases due to artificial intelligence?
Biases due to artificial intelligence can include gender bias, racial bias, age bias, and more. These biases can manifest in various AI applications and impact decision-making processes, potentially leading to unfair treatment or outcomes.
6. What is the gender of AI?
AI does not have a gender, as it is a technology created by humans. However, gendered characteristics or biases may be inadvertently encoded into AI systems through the data used to train them or the design choices made by developers.
7. What is the gender diversity in AI?
Gender diversity in AI refers to the representation of individuals of different genders in the field of artificial intelligence. These include researchers, developers, and practitioners.
Efforts are being made to increase gender diversity in AI to ensure a broader range of perspectives and experiences are considered in AI development.
8. Why is there gender bias in AI?
Gender bias in AI often stems from the biases present in the data used to train AI models and the decisions made by developers during the design and development process.
Biases in training data, such as historical inequalities and stereotypes, can be inadvertently learned by AI algorithms, leading to biased outcomes.
Additionally, human developers may unconsciously introduce biases into AI systems through their own beliefs, perspectives, and decision-making processes.
Lack of diversity in the AI development community can also contribute to gender bias. Yes, because diverse perspectives are essential for identifying and mitigating biases effectively.
Overall, gender bias in AI is a complex issue influenced by societal norms, historical inequalities, and the human factors involved in AI development.