AI hallucination is a fascinating phenomenon where a large language model (LLM), such as a generative AI chatbot or computer vision tool, perceives patterns or objects that are non-existent or imperceptible to human observers. This leads to the creation of outputs that are nonsensical or altogether inaccurate.
In the context of virtual reality, AI hallucinations play a significant role in creating realistic virtual experiences. The growing interest in AI hallucination and its potential impact on various industries has sparked the need to unravel the illusion of virtual reality created by these hallucinations.
The Implications of AI Hallucinations
AI hallucinations can have both positive and negative implications. On one hand, they can enhance virtual reality experiences by adding depth and realism. On the other hand, they can lead to misinformation, confusion, and even safety risks if not properly managed.
Addressing AI Hallucinations
To address AI hallucinations, researchers and developers are exploring techniques such as:
- Improving the quality and diversity of training data
- Refining the AI models
- Implementing human oversight and fact-checking
However, solving AI hallucinations entirely remains a complex challenge.
AI Hallucination: Exploring a Fascinating Phenomenon
AI hallucination is a fascinating phenomenon that occurs when AI systems attempt to “fill in the gaps” of their knowledge by generating content that meets the needs of the prompter. It is closely connected to generative AI, which utilizes deep learning algorithms to create realistic virtual experiences. These algorithms analyze vast amounts of data and learn patterns to generate outputs, even if the information isn’t accurate or factual.
The root cause of AI hallucinations lies in the limitations of the training data and the model’s ability to interpret and generate content. Insufficient or biased training data can lead to AI systems fabricating details or generating false information. Overfitting, where the model becomes too focused on the details and noise in the training data, can also contribute to hallucinations. Additionally, faulty model assumptions or architecture can impact the model’s ability to accurately interpret data.
The Impact of AI Hallucinations
An example of AI hallucination can be seen in the context of chatbots. ChatGPT, for instance, has been known to generate responses that are factually incorrect or misleading. This can range from fabricated information to strange and creepy responses, and even harmful misinformation. These hallucinations can have serious consequences, such as the spread of disinformation and misinformation, lowered user trust, and safety risks.
Dealing with Hallucinations in Generative AI
Dealing with hallucinations in generative AI requires a multi-faceted approach. Here are some strategies:
- Curate high-quality and diverse training data: Ensure that AI models are exposed to accurate and representative samples of the target domain. Incorporate a wide range of inputs, including various styles, genres, or perspectives, to foster the generation of imaginative yet coherent content.
- Prompt engineering and model refinement: Use clear and specific prompts grounded with relevant information and sources to guide the AI model in generating accurate responses. Experiment with temperature, which controls the randomness of the generated output, to influence the hallucination effect.
- Human fact-checking: Human reviewers play a crucial role in identifying and correcting inaccuracies that AI may overlook. Their expertise serves as an essential safeguard against AI hallucinations.
The Road to Improvement
AI hallucination is a sign that we are still in the early stages of AI development, and there is room for improvement. Efforts to improve data quality, refine models, and incorporate human oversight can help minimize the risks associated with AI hallucinations. By understanding the limitations of AI systems and implementing appropriate measures, we can harness the potential of AI while ensuring the generation of accurate and reliable content.
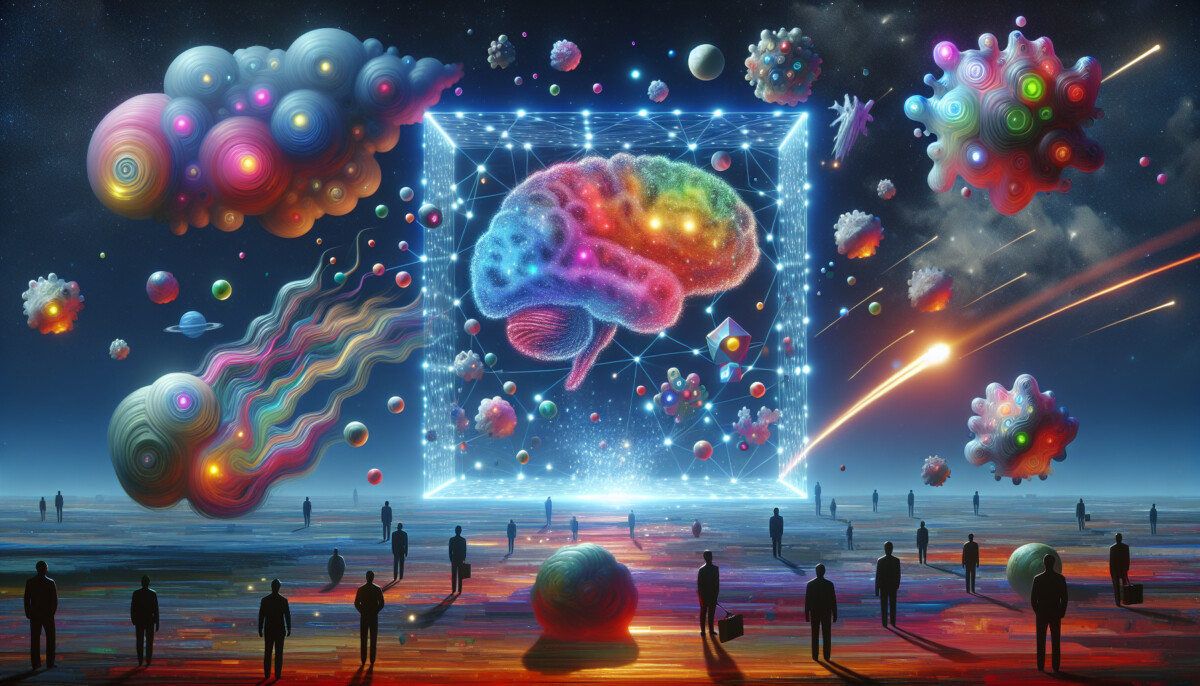
AI Hallucinations: Exploring Immersive Experiences and Potential Drawbacks
AI hallucinations have become increasingly prevalent in various industries, including entertainment, gaming, and virtual reality applications. In these fields, AI-generated characters and scenes have the potential to create immersive experiences for viewers. For example, AI-powered video games can generate lifelike characters and realistic environments, enhancing the overall gaming experience. AI-generated content in movies and TV shows can also provide visually stunning and captivating visuals.
However, it is important to recognize that AI hallucinations also come with potential drawbacks. One major concern is the dissemination of fake or misleading information. AI models, such as chatbots or language models, can generate false or inaccurate content that may be presented as factual information. This poses a challenge for users who rely on AI-generated content for research or decision-making purposes. Fact-checking becomes crucial in order to verify the accuracy of information provided by AI systems.
To address this issue, efforts are being made to improve the quality and diversity of the data used to train AI models. High-quality and diverse training data ensure that AI models are exposed to accurate and representative samples of the target domain, enhancing their ability to generate coherent and reliable content. Incorporating a wide range of inputs, including various styles, genres, or perspectives, exposes the models to a rich spectrum of information and encourages the generation of imaginative yet accurate content.
Despite the potential risks associated with AI hallucinations, it is important to view them as a sign that we are still in the early stages of AI development. AI hallucinations can be seen as external imagination that can help us think of new possibilities and ideas. By understanding the limitations of AI systems and implementing strategies to prevent hallucinations, we can harness the benefits of AI while minimizing the risks.
AI hallucinations have been utilized in various industries to create immersive experiences for users. However, they also raise concerns about the dissemination of fake or misleading information. Fact-checking and improving the quality of training data are important steps in addressing this issue. AI hallucinations should be viewed as an opportunity to reimagine the world and explore new possibilities while being mindful of the limitations of AI systems.
Factors contributing to AI hallucinations
AI hallucinations are a fascinating yet complex phenomenon that can be caused by several factors. One of the key contributors to the creation of AI hallucinations is the quality and diversity of the training data.
Training data quality: The training data is crucial in generating accurate outputs, as it forms the basis for the AI model’s understanding of the world. When the training data is biased or incomplete, it can lead to hallucinations where the AI model generates false or misleading information as if it were fact.
- Efforts are made to curate high-quality and diverse training datasets to ensure the generation of accurate and representative outputs.
- High-quality data ensures that AI models are exposed to accurate and reliable samples from the target domain, enhancing their ability to generate coherent hallucinations.
- Incorporating a wide range of inputs, including various styles, genres, or perspectives, exposes the models to a rich spectrum of information and encourages the generation of imaginative content.
- Scientific studies have shown that incorporating diverse data leads to enhanced creativity and the ability to produce more compelling and surprising hallucinations.
AI model design and training process: Apart from the training data, the design of the AI model and the process of training algorithms and neural networks also play a significant role in the generation of AI hallucinations. The design of the AI model, including its architecture and assumptions, can contribute to the model’s ability to interpret data correctly.
- Faulty model assumptions or architecture can lead to hallucinations as the AI model tries to fill in the gaps in its knowledge.
AI Hallucinations: Ethical Dilemmas
AI hallucinations raise a number of ethical dilemmas that need to be addressed. One of the main concerns is the potential for misinformation and manipulation. AI models, such as generative language models like ChatGPT, can generate false or misleading information as if it were factual. This can have serious consequences, as false information can spread quickly and have far-reaching impacts.
Potential Consequences
Reputational Harm
The spread of false information can lead to reputational harm for individuals or businesses. If AI hallucinations generate fabricated information about a person or company, it can damage their reputation and credibility. This can be particularly concerning for public figures, organizations, and businesses that rely on trust and accurate information to maintain their image.
Safety Risks
In addition to reputational harm, AI hallucinations can also pose safety risks. If AI models provide inaccurate or misleading information in critical situations, it can lead to harmful outcomes. For example, if a healthcare AI model hallucinates and provides incorrect medical advice, it could potentially endanger someone’s health or even cost lives.
Privacy and Consent Concerns
Privacy and consent concerns also arise when AI hallucinations gather and process personal data. AI models often require large amounts of data to learn and generate content. However, the use of personal data raises questions about consent and privacy. Users may not be aware of how their data is being used or may not have given explicit consent for its use in AI hallucinations.
Addressing the Challenges
To address these challenges, regulations and guidelines are necessary to ensure responsible use of AI hallucinations. It is important to establish ethical standards and guidelines for AI developers and organizations using AI models. This includes transparency in how AI models are trained, ensuring data privacy and consent, and implementing safeguards to prevent the spread of false or harmful information.
Additionally, efforts should be made to improve the quality and diversity of the data used to train AI models. High-quality data that is free from biases, errors, and inconsistencies is crucial for generating accurate and coherent hallucinations. Incorporating diverse data from various sources and perspectives can enhance the creativity and reliability of AI models.
Designing Solutions
To minimize the risk of creating misleading or harmful AI hallucinations, strategies can be implemented in the design of AI systems. Transparency, explainability, and user feedback are important factors in improving AI hallucination technology. Human oversight and accountability also play a vital role in mitigating the negative effects of AI hallucinations.
Transparency and Explainability
One of the key strategies in designing AI systems to minimize the risk of AI hallucinations is to prioritize transparency and explainability. When AI systems generate content, it’s crucial to provide users with information about how the AI arrived at its conclusions or responses. By understanding the underlying processes and algorithms, users can better evaluate and interpret the output. This transparency also helps build trust and confidence in AI technology.
User Feedback
Additionally, user feedback is essential in improving AI hallucination technology. By collecting feedback from users, developers can identify and address potential issues or biases in the AI system. User feedback can also help in fine-tuning the AI model to generate more accurate and reliable responses. Regularly updating and refining the AI system based on user feedback ensures that it aligns with users’ expectations and requirements.
Human Oversight and Accountability
Human oversight and accountability are critical in mitigating the negative effects of AI hallucinations. While AI systems can automate many tasks, it’s essential to have human reviewers and moderators who can review and validate the content generated by the AI. Human oversight acts as a safeguard to catch any inaccuracies, biases, or harmful information that the AI may produce. It also ensures that the AI system adheres to ethical standards and guidelines.
An example of the importance of designing solutions to minimize AI hallucinations can be seen in ChatGPT, a popular language model. ChatGPT has been known to produce hallucinations, such as generating false or inaccurate information. To address this issue, OpenAI, the organization behind ChatGPT, has implemented a moderation system that involves human reviewers checking and rating the model’s outputs. This human oversight helps improve the quality of the AI-generated content and reduces the risk of AI hallucinations.
Advancements in AI Hallucination Technology
Advancements in AI hallucination technology are continuously emerging, and these advancements have the potential to revolutionize virtual reality experiences in fields like healthcare, education, and communication. AI hallucinations refer to the phenomenon where chatbots or other tools based on large language models (LLMs) generate false or misleading information as fact. However, it is crucial to address concerns and evaluate the potential benefits of AI hallucinations in shaping the future.
AI hallucinations occur due to various factors, including:
- Overfitting
- Biased or incomplete training data
- Attack prompts
- Use of slang or idioms in prompts
The AI models behind these hallucinations learn by analyzing large amounts of data gathered online and use language patterns to create outputs, rather than understanding the meaning behind the words. This can lead to fabricated information, factual inaccuracy, weird and creepy responses, and harmful misinformation.
Preventing AI Hallucinations
To prevent AI hallucinations, users can take several steps:
- Write clear and to-the-point prompts to guide the AI model in generating accurate responses.
- Use multiple steps in prompting and ground prompts with relevant information and sources to improve the accuracy of AI-generated content.
- Establish constraints and rules, specifying what users want and don’t want the AI to produce.
It is important to understand the limitations of AI systems and verify every piece of information they provide. Human fact-checking remains one of the most effective safeguards against AI hallucinations, as human reviewers can identify and correct inaccuracies that AI may not recognize. Additionally, efforts should be made to use high-quality training data, implement structured data templates, and restrict the dataset to ensure accurate and reliable outputs.
Consequences of AI Hallucinations
AI hallucinations can have significant consequences for real-world applications. For example, fake legal quotes generated by AI models have been used in court cases, leading to misinformation and potentially influencing the outcome. In the healthcare industry, AI models may incorrectly identify benign skin lesions as malignant, leading to unnecessary treatments or delays in diagnosis.
Despite the potential risks, AI hallucinations can also be seen as a sign that we are still early in the development of this technology. They can be viewed as external imagination that can help us think of new possibilities and ideas. By recognizing the challenges and addressing the concerns associated with AI hallucinations, we can harness the power of AI technology while minimizing the risks.
Advancements in AI hallucination technology have the potential to revolutionize virtual reality experiences in various fields. However, it is important to address concerns and evaluate the potential benefits of AI hallucinations.
By taking steps to prevent AI hallucinations, such as writing clear prompts and implementing human fact-checking, we can ensure the accuracy and reliability of AI-generated content. By understanding the limitations and risks associated with AI hallucinations, we can harness the power of AI technology while minimizing potential negative consequences.
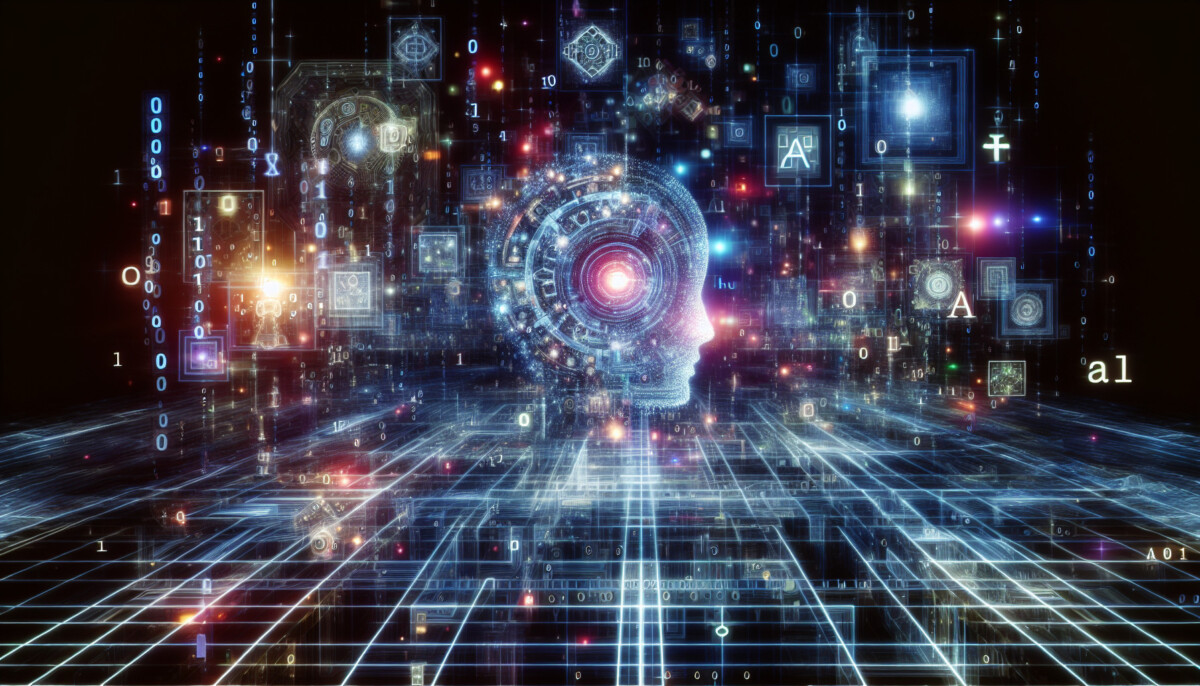
AI Hallucinations Unmasked: Unraveling the Illusion of Virtual Reality
In the realm of virtual reality, AI hallucinations present both opportunities and challenges. They have the potential to create immersive and realistic experiences, taking virtual reality to new heights. However, they also raise ethical considerations and the need for responsible use. By understanding the causes, challenges, and potential solutions surrounding AI hallucinations, we can navigate the complex landscape of virtual reality and ensure a balanced approach to their development and use.
What are AI Hallucinations?
AI hallucinations occur when AI systems generate false or misleading information as fact. These hallucinations can take various forms:
- Fabricated information and factual inaccuracy
- Weird and creepy responses
- Harmful misinformation
AI models attempt to “fill in the gaps” of their knowledge by creating content to meet the needs of the prompter. While generative AI is great at knowing complex information, it may not always know the bounds of that knowledge. Larger datasets provide a broader context for learning and allow the models to capture more nuanced patterns. However, the quality and diversity of the data used during training play a crucial role in the generation of AI hallucinations.
High-quality data ensures that AI models are exposed to accurate and representative samples of the target domain, enhancing their ability to generate coherent hallucinations. Incorporating a wide range of inputs, including various styles, genres, or perspectives, exposes the models to a rich spectrum of information and encourages the generation of imaginative content.
Scientific studies have shown that incorporating diverse data leads to enhanced creativity and the ability to produce more compelling and surprising hallucinations. Increasing the volume and quality of the data used to train AI may result in fewer erroneous fabrications and unlock greater depths of creativity.
Strategies to Deal with AI Hallucinations
To deal with hallucinations in generative AI, several strategies can be employed:
- Write clear and to-the-point prompts
- Use multiple steps in prompting
- Ground prompts with relevant information and sources
- Establish constraints and rules
- Specify what you want and don’t want the AI to produce
It is important to understand the limitations of AI systems and verify every piece of information they provide. Human fact-checking remains one of the most effective safeguards against AI hallucinations, as human reviewers can identify and correct inaccuracies that AI may not recognize. Additionally, prompt engineering and model refinement are two major ways to prevent AI hallucinations.
Prompt engineering techniques include using clear prompts, providing relevant information, and giving a role to the model. Model refinement techniques involve using diverse and relevant data, experimenting with temperature, and fine-tuning the model on the domain area. Detecting AI hallucinations is a complex task that sometimes requires field experts to fact-check the generated content. The collaborative effort between developers, researchers, and field experts is crucial in advancing solutions to minimize the risk of AI hallucinations.
Consequences and Risks of AI Hallucinations
An example of a hallucination in ChatGPT is when it incorrectly claims that the James Webb Space Telescope has captured the world’s first images of a planet outside our solar system. Such hallucinations can have significant consequences for real-world applications. For instance, in healthcare, AI models may incorrectly identify benign skin lesions as malignant, leading to incorrect diagnoses. In the legal field, AI-generated fake legal quotes can be used in court cases, resulting in misleading information being presented as evidence. It is essential to recognize the potential risks and challenges associated with AI hallucinations and take proactive measures to address them.
In conclusion, AI hallucinations in the realm of virtual reality offer exciting possibilities but also come with ethical considerations and the need for responsible use. By understanding the causes and challenges surrounding AI hallucinations, we can work towards developing solutions that minimize their occurrence and ensure the generation of accurate and reliable content. The development and use of AI in virtual reality should be approached with caution, taking into account the potential risks and the importance of human oversight. With a balanced and responsible approach, AI hallucinations can be managed effectively, leading to a more immersive and trustworthy virtual reality experience.
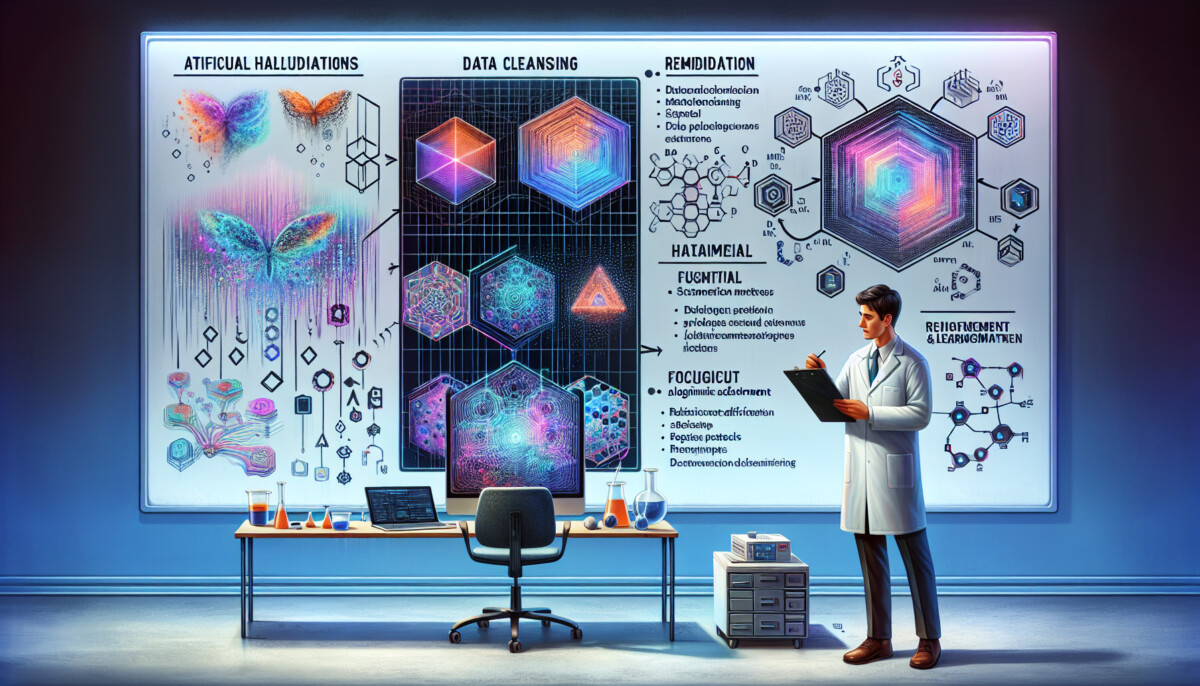
AI Hallucinations: Exploring the Phenomenon
AI hallucinations in generative AI have emerged as a fascinating yet complex phenomenon. As we explored in this blog, AI hallucinations occur when language models, like ChatGPT, generate false or misleading information that appears to be factual. These hallucinations can range from fabricated details to factual inaccuracies and even creepy or harmful responses.
But can AI hallucinations be solved? While there is no simple answer, efforts are being made to address this challenge. One key factor is the quality and diversity of the training data used. By curating high-quality, diverse datasets and incorporating a wide range of inputs, AI models can be exposed to accurate and representative samples, reducing the likelihood of hallucinations. Additionally, prompt engineering and model refinement techniques can help prevent AI hallucinations by providing clear prompts, relevant information, and refining the models on specific domains.
As we delve deeper into the world of generative AI, it’s important to understand the limitations and risks associated with AI hallucinations. However, these hallucinations can also be seen as external imagination that can help us think of new possibilities and ideas. By combining human oversight with AI technology, we can harness the creative potential of AI while minimizing the risks.